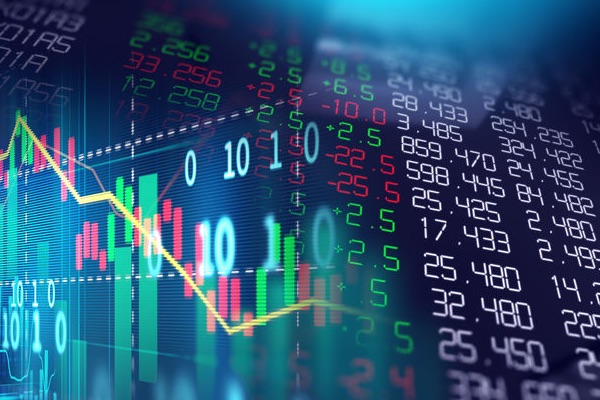
Today’s digital business makes data no longer just the exhaust emanating from operational systems. It is an essential ingredient to every business, underpinning decision making on everything from your customers and sales, to finance and support. Your ability to harness this data of ever-growing volume, variety, and velocity is at the heart of your future growth.
However, efficiently managing data pipelines is not easy. You most probably already ran into that situation where the business is asking for reports that need new data sources. And like many other organizations, you need weeks to integrate additional sources of data into your analytics pipeline. Often data is generated in too many places and stored in too many different silos. Many businesses struggle with this, creating synchronization issues, which in turn make it extremely difficult to guarantee data consistency. This explains why many IT organizations are still spending between 60% to 80% of their time getting the data rather than using the data. With Artificial Intelligence becoming a growing enterprise priority, data preparation and sourcing is expected to become a more significant pain point, impeding the ability to train Machine Learning algorithms to attain trust the models must provide.
To fully leverage the benefits of AI and ML, you have to adapt your analytics processes and data flows to move beyond the traditional data warehouse silos. The idea is to better integrate analytics developments with DevOps practices, where building, testing, provisioning, and deploying are all run as automated processes. Emerging disciplines, like DataOps, incorporate agile approaches to minimize the cycle time of analytics development. They aim to orchestrate environments, tools, models, and data from an end-to-end perspective, bringing together Data Scientists with the Operations teams improve the data lifecycle management and enabling a fully efficient data pipeline. Of course, putting DataOps to work requires addressing some specific organizational and automation challenges.
Integrating data-driven environments into DevOps
When you look at the vast number of new projects aimed to leverage the value of existing data, you quickly realize that many companies run Big Data environments in near-total isolation from the rest of enterprise business processes. Of course, you may find good reasons for that: Big Data is a new technology that requires new skills that involve new teams. But running Big Data as a silo prevents you from integrating data-driven environments into your enterprise DevOps initiatives. The reality is that your data pipelines have to be automated along your continuous delivery pipelines, and for that, you need automation that goes beyond silos.
Delivering the right data on time, all the time
But there is a further side effect of siloed data and disjointed automation. Data Scientists find data flows much too complex to design and manage, and they always require extended support from the IT experts. So, many IT organizations have difficulties in scaling with the volume of data, or the number of data sources, without slowing down development cycle times. As a result, innovation and customer experience are significantly impacted by the delays in getting access to data.
Keeping your data pipelines compliant
However, slow data delivery is not the only threat induced by poor visibility and control over your data pipelines. Manual operations and manual handovers are increasing the risks of compliance issues. Just think about how data protection regulations are thriving everywhere around the globe. To ensure you stay compliant with your data pipelines, you need to secure every operation, trace who’s doing what, and when he has been doing it.
Access to the right information at the right time is essential to succeed with your digital transformation initiatives, to get ahead of the competition, and drive continuous innovation. Cumbersome data sourcing and management do not fit into the new fast-changing business environment. Broadcom, who is committed to helping companies simplifying and accelerating the integration of Big Data initiatives with efficient automation and orchestration, has been referenced as a representative vendor in the latest “Market Guide for Service Orchestration and Automation Platforms (SOAP)” from Gartner. SOAP is a new category of automation solutions that can act as a single point of orchestration for data pipelines, resource provisioning, and self-service across on-prem, private, and public cloud environments. Broadcom’s solution enables workflows to be designed visually without coding, integrates with third-party tools, and scales up to thousands of data flows. Data Engineers and non-technical users like Data Scientists are empowered with self-service, reducing the effort from developers and platform experts, improving agility and compliance.
Enterprises are dealing with the increasing volume of structured and unstructured data. However, most organizations still do not have mature processes for converting data into insights that can help them to continuously reinvent their business. Modern automation can help industrializing data pipelines, increasing agility, reducing cycle times, and building a new level of trust in the use of ML and AI. This might be enough incentive for you to start reviewing your automation strategies and modernize your data pipelines.